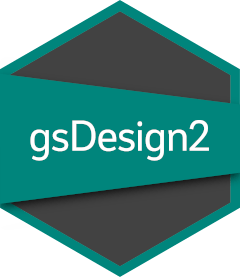
Canonical joint distribution of Z-score and B-values under null and alternative hypothesis
Yujie Zhao, Yilong Zhang, and Keaven M. Anderson
Source:vignettes/articles/story-canonical-h0-h1.Rmd
story-canonical-h0-h1.Rmd
When applying the AHR test in the time-to-event endpoint at the k-th analysis, we build the standardized treatment effect as the test statistics in Proschan, Lan, and Wittes (2006):
Z_k = \frac{ \sum_{i=1}^{d_k} \Delta_i }{ \sqrt{\text{Var}(\sum_{i=1}^{d_k} \Delta_i | H_0)} },
where d_k is the total number of events at analysis k. Here, \Delta_i = O_i - E_i with O_i is the indicator that the i-th event occurred in the experimental arm, and E_i = m_{i,1}/(m_{i,0} + m_{i,1}) as the null expectation of O_i given m_{i,0} and m_{i,1}. The numbers m_{i,0} and m_{i,1} refer to the number of at-risk subjects prior to the i-th death in the control and experimental group, respectively. When conditioning on m_{i,0} and m_{i,1}, the O_i has a Bernoulli distribution with parameter E_i. So the null conditional mean and variance of \Delta_i are 0 and V_i = E_i(1 - E_i), respectively. Unconditionally, \Delta_i are mean 0 random variables with variance E(V_i) under the null hypothesis. So, conditioned on d_k, we have \text{Var}(\sum_{i=1}^{d_k} \Delta_i | H_0)) = E(\sum_{i=1}^{d_k} V_i).
In this vignette, we discuss the statistical information. Mathematically, it is the inverse of the variance, depending on whether it is under null or alternative hypothesis:
\begin{array}{c} \mathcal I_{k, H_0} = 1 /\text{Var}(\sum_{i=1}^{d_k} \Delta_i | H_0), \\ \mathcal I_{k, H_1} = 1 /\text{Var}(\sum_{i=1}^{d_k} \Delta_i | H_1). \end{array}
The ratio between two statistical information under the null hypothesis is called information fraction:
t_k = \frac{\text{Var}(\sum_{i=1}^{d_k} \Delta_i | H_0)}{\text{Var}(\sum_{i=1}^{d_K} \Delta_i | H_0)} \approx \frac{d_k/4}{d_K / 4} = d_k /d_K,
In the rest of this vignette, we will discuss the value of \mathcal I_{k, H_0}, \mathcal I_{k, H_1}. To derive these statistical information, we introduce the B-values:
B_k = \frac{ \sum_{i=1}^{d_k} \Delta_i }{ \sqrt{\text{Var}(\sum_{i=1}^{d_K} \Delta_i | H_0)} } = \underbrace{ \frac{ \sum_{i=1}^{d_k} \Delta_i }{ \sqrt{\text{Var}(\sum_{i=1}^{d_k} \Delta_i | H_0)} } }_{Z_k} \underbrace{ \frac{ \sqrt{\text{Var}(\sum_{i=1}^{d_k} \Delta_i | H_0)} }{ \sqrt{\text{Var}(\sum_{i=1}^{d_K} \Delta_i | H_0)} } }_{\sqrt{t_k}} = \sqrt{t_k} Z_k,
As depicted in Section 2.1.3 of Proschan, Lan, and Wittes (2006), the stochastic process formulation presented in Harrington, Fleming, and Green (1984) and Tsiatis (1982) provides insights that sequence of B-values, denoted as {B_1, \ldots, B_K} with follows a multivariate normal distribution behaves asymptotically like Brownian motion.
For 2 B-values (B_i, B_j with i \leq j), we have
\begin{eqnarray} \text{Cov}(B_i, B_j) & = & \text{Cov} \left( \frac{ \sum_{s=1}^{d_i} \Delta_s }{ \sqrt{\text{Var}(\sum_{s=1}^{d_K} \Delta_s | H_0)} }, \frac{ \sum_{s=1}^{d_j} \Delta_s }{ \sqrt{\text{Var}(\sum_{s=1}^{d_K} \Delta_s | H_0)} } \right) \\ & = & \frac{1}{\text{Var}(\sum_{s=1}^{d_K} \Delta_s | H_0)} \text{Cov} \left( \sum_{s=1}^{d_i} \Delta_s, \sum_{s=1}^{d_j} \Delta_s \right) \\ & = & \frac{1}{\text{Var}(\sum_{s=1}^{d_K} \Delta_s | H_0)} \text{Cov} \left( \sum_{s=1}^{d_i} \Delta_s, \sum_{s=1}^{d_i} \Delta_s + \sum_{s=1}^{d_j} \Delta_s - \sum_{s=1}^{d_i} \Delta_s \right) \\ & = & \frac{1}{\text{Var}(\sum_{s=1}^{d_K} \Delta_s | H_0)} \text{Var} \left( \sum_{s=1}^{d_i} \Delta_s \right) + \text{Cov} \left( \sum_{s=1}^{d_i} \Delta_s, \sum_{s=1}^{d_j} \Delta_s - \sum_{s=1}^{d_i} \Delta_s \right) \\ & = & \frac{1}{\text{Var}(\sum_{s=1}^{d_K} \Delta_s | H_0)} \text{Var} \left( \sum_{s=1}^{d_i} \Delta_s \right) \end{eqnarray}
Null hypothesis
The distribution of \{B_k\}_{k = 1, \ldots, K} has the following structure:
- B_1, B_2, \ldots, B_K have a multivariate normal distribution.
- E(B_k \;|\; H_0) = 0 for any k = 1, \ldots, K.
- \text{Var}(B_k \;|\; H_0) = t_k.
- \text{Cov}(B_i, B_j \;|\; H_0) = t_i for any 1 \leq i \leq j \leq K.
The derivation of the last 2 statement is
\begin{eqnarray} \text{Var}(B_k\;|\; H_0) & = & \frac{ \text{Var}(\sum_{i=1}^{d_k} \Delta_i | H_0) }{ \text{Var}(\sum_{i=1}^{d_K} \Delta_i | H_0) } = t_k\\ \text{Cov}(B_i, B_j \;|\; H_0) & = & \frac{1}{\text{Var}(\sum_{s=1}^{d_K} \Delta_s\;|\; H_0)} \text{Var} \left( \sum_{s=1}^{d_i} \Delta_s\;|\; H_0 \right) = t_i \end{eqnarray}
Accordingly, \{Z_k\}_{k = 1, \ldots, K} has the canonical joint distribution with the following properties:
- Z_1, Z_2, \ldots, Z_K have a multivariate normal distribution.
- E(Z_k \;|\; H_0) = 0.
- \text{Var}(Z_k \;|\; H_0) = 1.
- \text{Cov}(Z_i, Z_j \;|\; H_0) = \sqrt{t_i/t_j} for any 1 \leq i \leq j \leq K.
Alternative hypothesis
Under the alternative hypothesis, for 2 B-values (B_i, B_j with i \leq j), the distribution of \{B_k\}_{k = 1, \ldots, K} has the following structure:
- B_1, B_2, \ldots, B_K have a multivariate normal distribution.
- E(B_k \;|\; H_1) = \theta_k t_k \sqrt{\mathcal I_{k, H_0}} for any k = 1, \ldots, K.
- \text{Var}(B_k \;|\; H_1) = t_k \mathcal I_{k, H_0} / \mathcal I_{k, H_1}.
- \text{Cov}(B_i, B_j \;|\; H_1) = t_i \; \mathcal I_{i, H_0}/\mathcal I_{i, H_1} for any 1 \leq i \leq j \leq K.
The last statement is derived as
\begin{eqnarray} \text{Cov}(B_i, B_j \;|\; H_1) & = & \frac{1}{\text{Var}(\sum_{s=1}^{d_K} \Delta_s | H_0)} \text{Var} \left( \sum_{s=1}^{d_i} \Delta_s | H_1 \right) \\ & = & \underbrace{ \frac{1}{\text{Var}(\sum_{s=1}^{d_K} \Delta_s | H_0)} \text{Var} \left( \sum_{s=1}^{d_i} \Delta_s | H_0 \right) }_{t_i} \underbrace{ \text{Var} \left( \sum_{s=1}^{d_i} \Delta_s | H_1 \right) }_{1/\mathcal I_{i, H_1}} \bigg/ \underbrace{ \text{Var} \left( \sum_{s=1}^{d_i} \Delta_s | H_0 \right) }_{1/\mathcal I_{i, H_0}} \\ & = & t_i\; \mathcal I_{i, H_0}/\mathcal I_{i, H_1}. \end{eqnarray}
Accordingly, Z_k has the canonical joint distribution with the following properties:
- Z_1, Z_2, \ldots, Z_K have a multivariate normal distribution.
- E(Z_k \;|\; H_1) = \theta_k \sqrt{\mathcal I_{k, H_0}} with the treatment effect as \theta_k at the k-th analysis.
- \text{Var}(Z_k \;|\; H_1) = \mathcal I_{k, H_0} / \mathcal I_{k, H_1}.
- \text{Cov}(Z_i, Z_j \;|\; H_1) = \sqrt{\frac{t_i}{t_j}} \frac{\mathcal I_{i, H_0}}{\mathcal I_{i, H_1}} for any 1 \leq i \leq j \leq K.
The last statement is because
\begin{eqnarray} \text{Cov}(Z_i, Z_j \;|\; H_1) & = & \text{Cov}(B_i/\sqrt{t_i}, B_j/\sqrt{t_j}) \\ & = & \frac{1}{\sqrt{t_i t_j}} \text{Cov}(B_i, B_j) \\ & = & \frac{1}{\sqrt{t_i t_j}} \text{Var}(B_i) \\ & = & \sqrt{\frac{t_i}{t_j}} \frac{\mathcal I_{i, H_0}}{\mathcal I_{i, H_1}} \end{eqnarray}
When the local alternative assumption holds, we have \text{Cov}(Z_i, Z_j) \approx \sqrt{\frac{t_i}{t_j}}, which is in the format of the canonical joint distribution introduced in Chapter 3 of Proschan, Lan, and Wittes (2006).
Summary
B-value | Z-score | |
---|---|---|
Expectation mean at the k-th analysis under H_0 | 0 | 0 |
Expectation mean at the k-th analysis under H_1 | \theta_k t_k \sqrt{\mathcal I_{k, H_0}} | \theta_k \sqrt{\mathcal I_{k, H_0}} |
Variance at the k-th analysis under H_0 | t_k | 1 |
Variance at the k-th analysis under H_1 | t_k \mathcal I_{k, H_0} / \mathcal I_{k, H_1} | \mathcal I_{k, H_0} / \mathcal I_{k, H_1} |
Covariance between the i-th and jth analysis under H_0 (i\leq j) | t_i | \sqrt{t_i/t_j} |
Covariance between the i-th and jth analysis under H_1 (i\leq j) | t_i \; \mathcal I_{i, H_0}/\mathcal I_{i, H_1} | \sqrt{\frac{t_i}{t_j}} \frac{\mathcal I_{i, H_0}}{\mathcal I_{i, H_1}} |