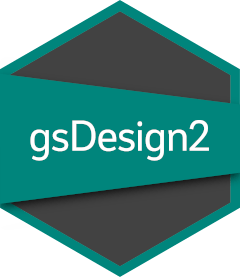
Group sequential design using average hazard ratio under non-proportional hazards
Source:R/gs_update_ahr.R
gs_update_ahr.Rd
Group sequential design using average hazard ratio under non-proportional hazards
Arguments
- x
A design created by either
gs_design_ahr()
orgs_power_ahr()
.- alpha
Type I error for the updated design.
- ustime
Default is NULL in which case upper bound spending time is determined by timing. Otherwise, this should be a vector of length k (total number of analyses) with the spending time at each analysis.
- lstime
Default is NULL in which case lower bound spending time is determined by timing. Otherwise, this should be a vector of length k (total number of analyses) with the spending time at each analysis.
- event_tbl
A data frame with two columns: (1) analysis and (2) event, which represents the events observed at each analysis per piecewise interval. This can be defined via the
pw_observed_event()
function or manually entered. For example, consider a scenario with two intervals in the piecewise model: the first interval lasts 6 months with a hazard ratio (HR) of 1, and the second interval follows with an HR of 0.6. The data frameevent_tbl = data.frame(analysis = c(1, 1, 2, 2), event = c(30, 100, 30, 200))
indicates that 30 events were observed during the delayed effect period, 130 events were observed at the IA, and 230 events were observed at the FA.
Examples
library(gsDesign)
library(gsDesign2)
library(dplyr)
alpha <- 0.025
beta <- 0.1
ratio <- 1
# Enrollment
enroll_rate <- define_enroll_rate(
duration = c(2, 2, 10),
rate = (1:3) / 3)
# Failure and dropout
fail_rate <- define_fail_rate(
duration = c(3, Inf), fail_rate = log(2) / 9,
hr = c(1, 0.6), dropout_rate = .0001)
# IA and FA analysis time
analysis_time <- c(20, 36)
# Randomization ratio
ratio <- 1
# ------------------------------------------------- #
# Two-sided asymmetric design,
# beta-spending with non-binding lower bound
# ------------------------------------------------- #
# Original design
x <- gs_design_ahr(
enroll_rate = enroll_rate, fail_rate = fail_rate,
alpha = alpha, beta = beta, ratio = ratio,
info_scale = "h0_info",
info_frac = NULL, analysis_time = c(20, 36),
upper = gs_spending_bound,
upar = list(sf = sfLDOF, total_spend = alpha),
test_upper = TRUE,
lower = gs_spending_bound,
lpar = list(sf = sfLDOF, total_spend = beta),
test_lower = c(TRUE, FALSE),
binding = FALSE) %>% to_integer()
planned_event_ia <- x$analysis$event[1]
planned_event_fa <- x$analysis$event[2]
# Updated design with 190 events observed at IA,
# where 50 events observed during the delayed effect.
# IA spending = observed events / final planned events, the remaining alpha will be allocated to FA.
gs_update_ahr(
x = x,
ustime = c(190 / planned_event_fa, 1),
lstime = c(190 / planned_event_fa, 1),
event_tbl = data.frame(analysis = c(1, 1),
event = c(50, 140)))
#> $enroll_rate
#> # A tibble: 3 × 3
#> stratum duration rate
#> <chr> <dbl> <dbl>
#> 1 All 2 10.6
#> 2 All 2 21.2
#> 3 All 10 31.8
#>
#> $fail_rate
#> # A tibble: 2 × 5
#> stratum duration fail_rate dropout_rate hr
#> <chr> <dbl> <dbl> <dbl> <dbl>
#> 1 All 3 0.0770 0.0001 1
#> 2 All Inf 0.0770 0.0001 0.6
#>
#> $bound
#> # A tibble: 4 × 7
#> analysis bound probability probability0 z `~hr at bound` `nominal p`
#> <int> <chr> <dbl> <dbl> <dbl> <dbl> <dbl>
#> 1 1 upper 0.481 0.00414 2.64 0.682 0.00414
#> 2 2 upper 0.906 0.0237 1.98 0.799 0.0237
#> 3 1 lower 0.0353 0.784 0.787 0.892 0.216
#> 4 2 lower 0.0353 0.784 -Inf Inf 1
#>
#> $analysis
#> analysis time n event ahr theta info info0 info_frac
#> 1 1 19.91897 382 190 0.6863292 0.3763978 47.50 47.50 0.6109325
#> 2 2 36.06513 382 311 0.6829028 0.3814027 77.75 77.75 1.0000000
#> info_frac0
#> 1 0.6109325
#> 2 1.0000000
#>
#> attr(,"class")
#> [1] "non_binding" "ahr" "gs_design" "list"
#> [5] "updated_design"
# Updated design with 190 events observed at IA, and 300 events observed at FA,
# where 50 events observed during the delayed effect.
# IA spending = observed events / final planned events, the remaining alpha will be allocated to FA.
gs_update_ahr(
x = x,
ustime = c(190 / planned_event_fa, 1),
lstime = c(190 / planned_event_fa, 1),
event_tbl = data.frame(analysis = c(1, 1, 2, 2),
event = c(50, 140, 50, 250)))
#> $enroll_rate
#> # A tibble: 3 × 3
#> stratum duration rate
#> <chr> <dbl> <dbl>
#> 1 All 2 10.6
#> 2 All 2 21.2
#> 3 All 10 31.8
#>
#> $fail_rate
#> # A tibble: 2 × 5
#> stratum duration fail_rate dropout_rate hr
#> <chr> <dbl> <dbl> <dbl> <dbl>
#> 1 All 3 0.0770 0.0001 1
#> 2 All Inf 0.0770 0.0001 0.6
#>
#> $bound
#> # A tibble: 4 × 7
#> analysis bound probability probability0 z `~hr at bound` `nominal p`
#> <int> <chr> <dbl> <dbl> <dbl> <dbl> <dbl>
#> 1 1 upper 0.481 0.00414 2.64 0.682 0.00414
#> 2 2 upper 0.939 0.0239 1.98 0.796 0.0238
#> 3 1 lower 0.0353 0.784 0.787 0.892 0.216
#> 4 2 lower 0.0353 0.784 -Inf Inf 1
#>
#> $analysis
#> analysis time n event ahr theta info info0 info_frac
#> 1 1 19.91897 382 190 0.6863292 0.3763978 47.5 47.5 0.6109325
#> 2 2 36.06513 382 300 0.6533201 0.4256880 75.0 75.0 1.0000000
#> info_frac0
#> 1 0.6333333
#> 2 1.0000000
#>
#> attr(,"class")
#> [1] "non_binding" "ahr" "gs_design" "list"
#> [5] "updated_design"
# Updated design with 190 events observed at IA, and 300 events observed at FA,
# where 50 events observed during the delayed effect.
# IA spending = minimal of planned and actual information fraction spending
gs_update_ahr(
x = x,
ustime = c(min(190, planned_event_ia) / planned_event_fa, 1),
lstime = c(min(190, planned_event_ia) / planned_event_fa, 1),
event_tbl = data.frame(analysis = c(1, 1, 2, 2),
event = c(50, 140, 50, 250)))
#> $enroll_rate
#> # A tibble: 3 × 3
#> stratum duration rate
#> <chr> <dbl> <dbl>
#> 1 All 2 10.6
#> 2 All 2 21.2
#> 3 All 10 31.8
#>
#> $fail_rate
#> # A tibble: 2 × 5
#> stratum duration fail_rate dropout_rate hr
#> <chr> <dbl> <dbl> <dbl> <dbl>
#> 1 All 3 0.0770 0.0001 1
#> 2 All Inf 0.0770 0.0001 0.6
#>
#> $bound
#> # A tibble: 4 × 7
#> analysis bound probability probability0 z `~hr at bound` `nominal p`
#> <int> <chr> <dbl> <dbl> <dbl> <dbl> <dbl>
#> 1 1 upper 0.481 0.00414 2.64 0.682 0.00414
#> 2 2 upper 0.939 0.0239 1.98 0.796 0.0238
#> 3 1 lower 0.0353 0.784 0.787 0.892 0.216
#> 4 2 lower 0.0353 0.784 -Inf Inf 1
#>
#> $analysis
#> analysis time n event ahr theta info info0 info_frac
#> 1 1 19.91897 382 190 0.6863292 0.3763978 47.5 47.5 0.6109325
#> 2 2 36.06513 382 300 0.6533201 0.4256880 75.0 75.0 1.0000000
#> info_frac0
#> 1 0.6333333
#> 2 1.0000000
#>
#> attr(,"class")
#> [1] "non_binding" "ahr" "gs_design" "list"
#> [5] "updated_design"
# Alpha is updated to 0.05
gs_update_ahr(x = x, alpha = 0.05)
#> $enroll_rate
#> # A tibble: 3 × 3
#> stratum duration rate
#> <chr> <dbl> <dbl>
#> 1 All 2 10.6
#> 2 All 2 21.2
#> 3 All 10 31.8
#>
#> $fail_rate
#> # A tibble: 2 × 5
#> stratum duration fail_rate dropout_rate hr
#> <chr> <dbl> <dbl> <dbl> <dbl>
#> 1 All 3 0.0770 0.0001 1
#> 2 All Inf 0.0770 0.0001 0.6
#>
#> $bound
#> # A tibble: 4 × 7
#> analysis bound probability probability0 z `~hr at bound` `nominal p`
#> <int> <chr> <dbl> <dbl> <dbl> <dbl> <dbl>
#> 1 1 upper 0.518 0.0150 2.17 0.737 0.0150
#> 2 2 upper 0.933 0.0487 1.69 0.826 0.0455
#> 3 1 lower 0.0413 0.684 0.478 0.935 0.316
#> 4 2 lower 0.0413 0.684 -Inf Inf 1
#>
#> $analysis
#> analysis time n event ahr theta info info0 info_frac
#> 1 1 19.91897 382 202 0.7322996 0.3115656 50.50 50.50 0.6495177
#> 2 2 36.06513 382 311 0.6829028 0.3814027 77.75 77.75 1.0000000
#> info_frac0
#> 1 0.6495177
#> 2 1.0000000
#>
#> attr(,"class")
#> [1] "non_binding" "ahr" "gs_design" "list"
#> [5] "updated_design"