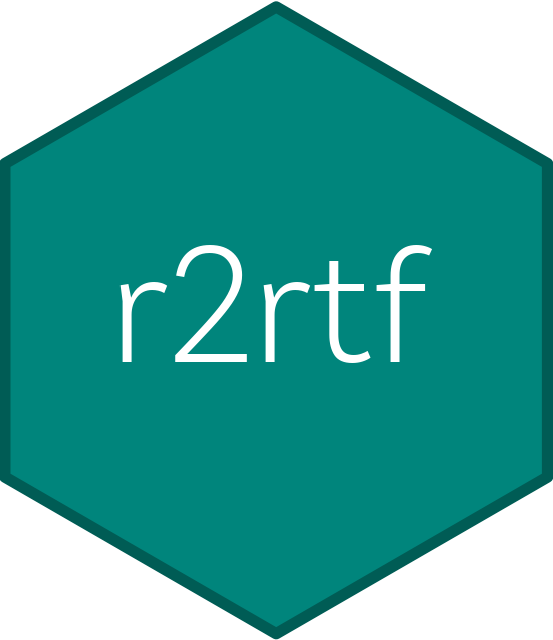
RTF Examples for Baseline Characteristics Tables
Source:vignettes/example-basechar.Rmd
example-basechar.Rmd
Example
This example shows how to create a baseline characteristic table as below.
Step 1: Define utility functions
We defined two utility functions for analysis purpose.
- Summarize categorical variable
bs_count <- function(data, grp, var,
var_label = var,
decimal = 1,
total = TRUE) {
data <- data %>% rename(grp = !!grp, var = !!var)
coding <- levels(factor(data$grp))
data <- data %>% mutate(grp = as.numeric(factor(grp)))
res <- with(data, table(var, grp)) %>%
as.data.frame() %>%
mutate(grp = as.numeric(grp))
if (total) {
res_tot <- with(data, table(var)) %>%
as.data.frame() %>%
mutate(grp = 9999)
res <- bind_rows(res, res_tot)
}
res <- res %>% rename(n = Freq)
res <- res %>% mutate(pct = formatC(n / sum(n) * 100, digits = decimal, format = "f", flag = "0"))
res$n <- as.character(res$n)
res <- res %>%
pivot_longer(cols = c(n, pct), names_to = "key", values_to = "value") %>%
unite(keys, grp, key) %>%
pivot_wider(names_from = keys, values_from = value) %>%
mutate(var_label = var_label) %>%
mutate(var = as.character(var))
names(res) <- gsub("_n", "", names(res), fixed = TRUE)
attr(res, "coding") <- coding
res
}
- Summarize continuous variable
bs_continuous <- function(data, grp, var,
var_label = var,
decimal = 1,
total = TRUE,
blank_row = FALSE) {
data <- data %>% rename(grp = !!grp, var = !!var)
coding <- levels(factor(data$grp))
data <- data %>% mutate(grp = as.numeric(factor(grp)))
res <- data %>%
select(grp, var) %>%
na.omit() %>%
group_by(grp) %>%
summarise(
`Subjects with data` = n(),
Mean = formatC(mean(var), digits = decimal, format = "f", flag = "0"),
SD = formatC(sd(var), digits = decimal, format = "f", flag = "0"),
Median = formatC(median(var), digits = decimal, format = "f", flag = "0"),
Range = paste(range(var), collapse = " to ")
)
if (total) {
res_tot <- data %>%
select(grp, var) %>%
na.omit() %>%
summarise(
`Subjects with data` = n(),
Mean = formatC(mean(var), digits = decimal, format = "f", flag = "0"),
SD = formatC(sd(var), digits = decimal, format = "f", flag = "0"),
Median = formatC(median(var), digits = decimal, format = "f", flag = "0"),
Range = paste(range(var), collapse = " to ")
) %>%
mutate(grp = 9999)
res <- bind_rows(res, res_tot)
}
res$"Subjects with data" <- as.character(res$"Subjects with data")
res <- res %>%
pivot_longer(cols = -grp, names_to = "key", values_to = "value") %>%
mutate(key = factor(key, levels = c("Subjects with data", "Mean", "SD", "Median", "Range"))) %>%
pivot_wider(names_from = grp, values_from = value) %>%
mutate(var_label = var_label) %>%
mutate(key = as.character(key)) %>%
rename(var = key)
if (blank_row) {
res <- bind_rows(tibble(var_label = var_label), res)
}
res
}
Step 2: Create data for rtf table
- Convert variable to factors to properly define display order
# The code above define two utility function for baseline characteristic tables.
# Analysis Set
data(r2rtf_adsl)
ana <- r2rtf_adsl %>% subset(ITTFL == "Y")
ana <- ana %>% mutate(
RACE = factor(
RACE,
c("WHITE", "BLACK OR AFRICAN AMERICAN", "AMERICAN INDIAN OR ALASKA NATIVE"),
c("White", "Black", "Other")
),
SEX = factor(
SEX,
c("F", "M"),
c("Female", "Male")
),
AGEGR1 = factor(
AGEGR1,
levels = c("<65", "65-80", ">80")
)
)
# Build Data for r2rtf
bs_tb <- bind_rows(
bs_count(ana, "TRT01AN", "SEX", "Gender"),
bs_count(ana, "TRT01AN", "AGEGR1", "Age (Years)"),
bs_continuous(ana, "TRT01AN", "AGE", "Age (Years)", blank_row = TRUE),
bs_count(ana, "TRT01AN", "RACE", "Race")
) %>% mutate(
var_label = factor(var_label, levels = c("Gender", "Age (Years)", "Race"))
)
bs_tb[is.na(bs_tb)] <- "" # convert NA to blank string.
knitr::kable(bs_tb)
var | 1 | 1_pct | 2 | 2_pct | 3 | 3_pct | 9999 | 9999_pct | var_label |
---|---|---|---|---|---|---|---|---|---|
Female | 53 | 10.4 | 50 | 9.8 | 40 | 7.9 | 143 | 28.1 | Gender |
Male | 33 | 6.5 | 34 | 6.7 | 44 | 8.7 | 111 | 21.9 | Gender |
<65 | 14 | 2.8 | 8 | 1.6 | 11 | 2.2 | 33 | 6.5 | Age (Years) |
65-80 | 42 | 8.3 | 47 | 9.3 | 55 | 10.8 | 144 | 28.3 | Age (Years) |
>80 | 30 | 5.9 | 29 | 5.7 | 18 | 3.5 | 77 | 15.2 | Age (Years) |
Age (Years) | |||||||||
Subjects with data | 86 | 84 | 84 | 254 | Age (Years) | ||||
Mean | 75.2 | 75.7 | 74.4 | 75.1 | Age (Years) | ||||
SD | 8.6 | 8.3 | 7.9 | 8.2 | Age (Years) | ||||
Median | 76.0 | 77.5 | 76.0 | 77.0 | Age (Years) | ||||
Range | 52 to 89 | 51 to 88 | 56 to 88 | 51 to 89 | Age (Years) | ||||
White | 78 | 15.4 | 78 | 15.4 | 74 | 14.6 | 230 | 45.3 | Race |
Black | 8 | 1.6 | 6 | 1.2 | 9 | 1.8 | 23 | 4.5 | Race |
Other | 0 | 0.0 | 0 | 0.0 | 1 | 0.2 | 1 | 0.2 | Race |
Step 3: Define table format
- page_by feature was used to display 3 parts (Gender, Age, Race)
clearly
- if using
new_page = TRUE
inrtb_body()
, the 3 parts will be outputted into 3 pages -
page_by
variable should not be inrtf_colheader()
. However, it should be inrtf_body()
- if using
bs_rtf <- bs_tb %>%
rtf_page(width = 9.5) %>%
rtf_title("Demographic and Anthropometric Characteristics", "ITT Subjects") %>%
rtf_colheader(" | Placebo | Drug Low Dose | Drug High Dose | Total ",
col_rel_width = c(3, rep(2, 4))
) %>%
rtf_colheader(" | n | (%) | n | (%) | n | (%) | n | (%) ",
col_rel_width = c(3, rep(c(1.2, 0.8), 4)),
border_top = c("", rep("single", 8)),
border_left = c("single", rep(c("single", ""), 4))
) %>%
rtf_body(
page_by = "var_label",
col_rel_width = c(3, rep(c(1.2, 0.8), 4), 3),
text_justification = c("l", rep("c", 8), "l"),
text_format = c(rep("", 9), "b"),
border_left = c("single", rep(c("single", ""), 4), "single"),
border_top = c(rep("", 9), "single"),
border_bottom = c(rep("", 9), "single"),
) %>%
rtf_footnote("This is a footnote") %>%
rtf_source("Source: xxx")
Step 4: Output
# Output .rtf file
bs_rtf %>%
rtf_encode() %>%
write_rtf("rtf/bs_example.rtf")