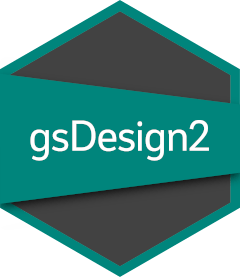
Group sequential design of binary outcome measuring in risk difference
Source:R/gs_design_rd.R
gs_design_rd.Rd
Group sequential design of binary outcome measuring in risk difference
Usage
gs_design_rd(
p_c = tibble::tibble(stratum = "All", rate = 0.2),
p_e = tibble::tibble(stratum = "All", rate = 0.15),
info_frac = 1:3/3,
rd0 = 0,
alpha = 0.025,
beta = 0.1,
ratio = 1,
stratum_prev = NULL,
weight = c("unstratified", "ss", "invar"),
upper = gs_b,
lower = gs_b,
upar = gsDesign(k = 3, test.type = 1, sfu = sfLDOF, sfupar = NULL)$upper$bound,
lpar = c(qnorm(0.1), rep(-Inf, 2)),
test_upper = TRUE,
test_lower = TRUE,
info_scale = c("h0_h1_info", "h0_info", "h1_info"),
binding = FALSE,
r = 18,
tol = 1e-06,
h1_spending = TRUE
)
Arguments
- p_c
Rate at the control group.
- p_e
Rate at the experimental group.
- info_frac
Statistical information fraction.
- rd0
Treatment effect under super-superiority designs, the default is 0.
- alpha
One-sided Type I error.
- beta
Type II error.
- ratio
Experimental:Control randomization ratio (not yet implemented).
- stratum_prev
Randomization ratio of different stratum. If it is unstratified design then
NULL
. Otherwise it is a tibble containing two columns (stratum and prevalence).- weight
The weighting scheme for stratified population.
- upper
Function to compute upper bound.
- lower
Function to compute lower bound.
- upar
Parameters passed to
upper
.- lpar
Parameters passed to
lower
.- test_upper
Indicator of which analyses should include an upper (efficacy) bound; single value of
TRUE
(default) indicates all analyses; otherwise, a logical vector of the same length asinfo
should indicate which analyses will have an efficacy bound.- test_lower
Indicator of which analyses should include an lower bound; single value of
TRUE
(default) indicates all analyses; single value ofFALSE
indicates no lower bound; otherwise, a logical vector of the same length asinfo
should indicate which analyses will have a lower bound.- info_scale
Information scale for calculation. Options are:
"h0_h1_info"
(default): variance under both null and alternative hypotheses is used."h0_info"
: variance under null hypothesis is used."h1_info"
: variance under alternative hypothesis is used.
- binding
Indicator of whether futility bound is binding; default of
FALSE
is recommended.- r
Integer value controlling grid for numerical integration as in Jennison and Turnbull (2000); default is 18, range is 1 to 80. Larger values provide larger number of grid points and greater accuracy. Normally,
r
will not be changed by the user.- tol
Tolerance parameter for boundary convergence (on Z-scale).
- h1_spending
Indicator that lower bound to be set by spending under alternate hypothesis (input
fail_rate
) if spending is used for lower bound.
Examples
library(gsDesign)
# Example 1 ----
# unstratified group sequential design
x <- gs_design_rd(
p_c = tibble::tibble(stratum = "All", rate = .2),
p_e = tibble::tibble(stratum = "All", rate = .15),
info_frac = c(0.7, 1),
rd0 = 0,
alpha = .025,
beta = .1,
ratio = 1,
stratum_prev = NULL,
weight = "unstratified",
upper = gs_b,
lower = gs_b,
upar = gsDesign(k = 2, test.type = 1, sfu = sfLDOF, sfupar = NULL)$upper$bound,
lpar = c(qnorm(.1), rep(-Inf, 2))
)
y <- gs_power_rd(
p_c = tibble::tibble(stratum = "All", rate = .2),
p_e = tibble::tibble(stratum = "All", rate = .15),
n = tibble::tibble(stratum = "All", n = x$analysis$n, analysis = 1:2),
rd0 = 0,
ratio = 1,
weight = "unstratified",
upper = gs_b,
lower = gs_b,
upar = gsDesign(k = 2, test.type = 1, sfu = sfLDOF, sfupar = NULL)$upper$bound,
lpar = c(qnorm(.1), rep(-Inf, 2))
)
# The above 2 design share the same power with the same sample size and treatment effect
x$bound$probability[x$bound$bound == "upper" & x$bound$analysis == 2]
#> [1] 0.9
y$bound$probability[y$bound$bound == "upper" & y$bound$analysis == 2]
#> [1] 0.9
# Example 2 ----
# stratified group sequential design
gs_design_rd(
p_c = tibble::tibble(
stratum = c("biomarker positive", "biomarker negative"),
rate = c(.2, .25)
),
p_e = tibble::tibble(
stratum = c("biomarker positive", "biomarker negative"),
rate = c(.15, .22)
),
info_frac = c(0.7, 1),
rd0 = 0,
alpha = .025,
beta = .1,
ratio = 1,
stratum_prev = tibble::tibble(
stratum = c("biomarker positive", "biomarker negative"),
prevalence = c(.4, .6)
),
weight = "ss",
upper = gs_spending_bound, lower = gs_b,
upar = list(sf = gsDesign::sfLDOF, total_spend = 0.025, param = NULL, timing = NULL),
lpar = rep(-Inf, 2)
)
#> $input
#> $input$p_c
#> # A tibble: 2 × 2
#> stratum rate
#> <chr> <dbl>
#> 1 biomarker positive 0.2
#> 2 biomarker negative 0.25
#>
#> $input$p_e
#> # A tibble: 2 × 2
#> stratum rate
#> <chr> <dbl>
#> 1 biomarker positive 0.15
#> 2 biomarker negative 0.22
#>
#> $input$info_frac
#> [1] 0.7 1.0
#>
#> $input$rd0
#> [1] 0
#>
#> $input$alpha
#> [1] 0.025
#>
#> $input$beta
#> [1] 0.1
#>
#> $input$ratio
#> [1] 1
#>
#> $input$stratum_prev
#> # A tibble: 2 × 2
#> stratum prevalence
#> <chr> <dbl>
#> 1 biomarker positive 0.4
#> 2 biomarker negative 0.6
#>
#> $input$weight
#> [1] "ss"
#>
#> $input$upper
#> function (k = 1, par = list(sf = gsDesign::sfLDOF, total_spend = 0.025,
#> param = NULL, timing = NULL, max_info = NULL), hgm1 = NULL,
#> theta = 0.1, info = 1:3, efficacy = TRUE, test_bound = TRUE,
#> r = 18, tol = 1e-06)
#> {
#> if (length(test_bound) == 1 && k > 1) {
#> test_bound <- rep(test_bound, k)
#> }
#> if (!is.null(par$timing)) {
#> timing <- par$timing
#> }
#> else {
#> if (is.null(par$max_info)) {
#> timing <- info/max(info)
#> }
#> else {
#> timing <- info/par$max_info
#> }
#> }
#> if (!is.function(sf <- par$sf))
#> sf <- tryCatch(match.fun(sf), error = function(e) {
#> getExportedValue("gsDesign", sf)
#> })
#> spend <- sf(alpha = par$total_spend, t = timing, param = par$param)$spend
#> old_spend <- 0
#> for (i in 1:k) {
#> if (test_bound[i]) {
#> xx <- spend[i] - old_spend
#> old_spend <- spend[i]
#> spend[i] <- xx
#> }
#> else {
#> spend[i] <- 0
#> }
#> }
#> spend <- spend[k]
#> if (!efficacy) {
#> if (spend <= 0) {
#> return(-Inf)
#> }
#> if (length(theta) == 1)
#> theta <- rep(theta, length(info))
#> a <- qnorm(spend) + sqrt(info[k]) * theta[k]
#> if (k == 1) {
#> return(a)
#> }
#> mu <- theta[k] * sqrt(info[k])
#> extreme_low <- mu - 3 - 4 * log(r)
#> extreme_high <- mu + 3 + 4 * log(r)
#> adelta <- 1
#> j <- 0
#> while (abs(adelta) > tol) {
#> hg <- hupdate(theta = theta[k], info = info[k], a = -Inf,
#> b = a, thetam1 = theta[k - 1], im1 = info[k -
#> 1], gm1 = hgm1, r = r)
#> i <- length(hg$h)
#> pik <- sum(hg$h)
#> adelta <- spend - pik
#> dplo <- hg$h[i]/hg$w[i]
#> if (adelta > dplo) {
#> adelta <- 1
#> }
#> else if (adelta < -dplo) {
#> adelta <- -1
#> }
#> else {
#> adelta <- adelta/dplo
#> }
#> a <- a + adelta
#> if (a > extreme_high) {
#> a <- extreme_high
#> }
#> else if (a < extreme_low) {
#> a <- extreme_low
#> }
#> if (abs(adelta) < tol) {
#> return(a)
#> }
#> j <- j + 1
#> if (j > 20) {
#> stop(paste("gs_spending_bound(): bound_update did not converge for lower bound calculation, analysis",
#> k, " !"))
#> }
#> }
#> }
#> else {
#> if (spend <= 0) {
#> return(Inf)
#> }
#> if (length(theta) == 1)
#> theta <- rep(theta, length(info))
#> b <- qnorm(spend, lower.tail = FALSE)
#> if (k == 1) {
#> return(b)
#> }
#> mu <- theta[k] * sqrt(info[k])
#> extreme_low <- mu - 3 - 4 * log(r)
#> extreme_high <- mu + 3 + 4 * log(r)
#> bdelta <- 1
#> j <- 1
#> while (abs(bdelta) > tol) {
#> hg <- hupdate(theta = 0, info = info[k], a = b, b = Inf,
#> thetam1 = 0, im1 = info[k - 1], gm1 = hgm1, r = r)
#> pik <- sum(hg$h)
#> bdelta <- spend - pik
#> dpikdb <- hg$h[1]/hg$w[1]
#> if (bdelta > dpikdb) {
#> bdelta <- 1
#> }
#> else if (bdelta < -dpikdb) {
#> bdelta <- -1
#> }
#> else {
#> bdelta <- bdelta/dpikdb
#> }
#> b <- b - bdelta
#> if (b > extreme_high) {
#> b <- extreme_high
#> }
#> else if (b < extreme_low) {
#> b <- extreme_low
#> }
#> if (abs(bdelta) < tol) {
#> return(b)
#> }
#> j <- j + 1
#> if (j > 20) {
#> stop(paste("gs_spending_bound(): bound_update did not converge for lower bound calculation, analysis",
#> k, " !"))
#> }
#> }
#> }
#> }
#> <bytecode: 0x55e5506c1358>
#> <environment: namespace:gsDesign2>
#>
#> $input$upar
#> $input$upar$sf
#> function (alpha, t, param = NULL)
#> {
#> checkScalar(alpha, "numeric", c(0, Inf), c(FALSE, FALSE))
#> checkVector(t, "numeric", c(0, Inf), c(TRUE, FALSE))
#> if (is.null(param) || param < 0.005 || param > 20)
#> param <- 1
#> checkScalar(param, "numeric", c(0.005, 20), c(TRUE, TRUE))
#> t[t > 1] <- 1
#> if (param == 1) {
#> rho <- 1
#> txt <- "Lan-DeMets O'Brien-Fleming approximation"
#> parname <- "none"
#> }
#> else {
#> rho <- param
#> txt <- "Generalized Lan-DeMets O'Brien-Fleming"
#> parname <- "rho"
#> }
#> z <- -qnorm(alpha/2)
#> x <- list(name = txt, param = param, parname = parname, sf = sfLDOF,
#> spend = 2 * (1 - pnorm(z/t^(rho/2))), bound = NULL, prob = NULL)
#> class(x) <- "spendfn"
#> x
#> }
#> <bytecode: 0x55e549003bc0>
#> <environment: namespace:gsDesign>
#>
#> $input$upar$total_spend
#> [1] 0.025
#>
#> $input$upar$param
#> NULL
#>
#> $input$upar$timing
#> NULL
#>
#>
#> $input$test_upper
#> [1] TRUE
#>
#> $input$lower
#> function (par = NULL, k = NULL, ...)
#> {
#> if (is.null(k)) {
#> return(par)
#> }
#> else {
#> return(par[k])
#> }
#> }
#> <bytecode: 0x55e5506b8088>
#> <environment: namespace:gsDesign2>
#>
#> $input$lpar
#> [1] -Inf -Inf
#>
#> $input$test_lower
#> [1] TRUE
#>
#> $input$h1_spending
#> [1] TRUE
#>
#> $input$binding
#> [1] FALSE
#>
#> $input$info_scale
#> [1] "h0_h1_info"
#>
#> $input$r
#> [1] 18
#>
#> $input$tol
#> [1] 1e-06
#>
#>
#> $bound
#> # A tibble: 2 × 7
#> analysis bound probability probability0 z `~risk difference at bound`
#> <int> <chr> <dbl> <dbl> <dbl> <dbl>
#> 1 1 upper 0.616 0.00738 2.44 0.0339
#> 2 2 upper 0.900 0.0250 2.00 0.0232
#> # ℹ 1 more variable: `nominal p` <dbl>
#>
#> $analysis
#> # A tibble: 2 × 8
#> analysis n rd rd0 info info0 info_frac info_frac0
#> <int> <dbl> <dbl> <dbl> <dbl> <dbl> <dbl> <dbl>
#> 1 1 3426. 0.038 0 5184. 5172. 0.7 0.7
#> 2 2 4894. 0.038 0 7406. 7388. 1 1
#>
#> attr(,"class")
#> [1] "non_binding" "rd" "gs_design" "list"
#> attr(,"uninteger_is_from")
#> [1] "gs_design_rd"